Agent-Agent interfaces and Google's new A2A protocol
This week, Google announced (↗) their new Agent-to-Agent protocol, A2A, designed to standardise how AI agents collaborate, even when run by different organisations using different underlying models. Positioned as complementary to MCP – which standardises agent access to external tools – A2A aims to standardise direct agent-agent communication. Google even declared A2A ♥️ MCP (↗), highlighting their vision for synergy between these protocols.
At Portia, we’ve been thinking about how agents interact with external systems via tools and agents for some time. You may have even read our post two weeks ago, Software interfaces in the agent era (↗). We divided the topic of agent integration with external systems into five categories based on increasing complexity, and A2A sits firmly at the top, in the Agent-Agent interface level.
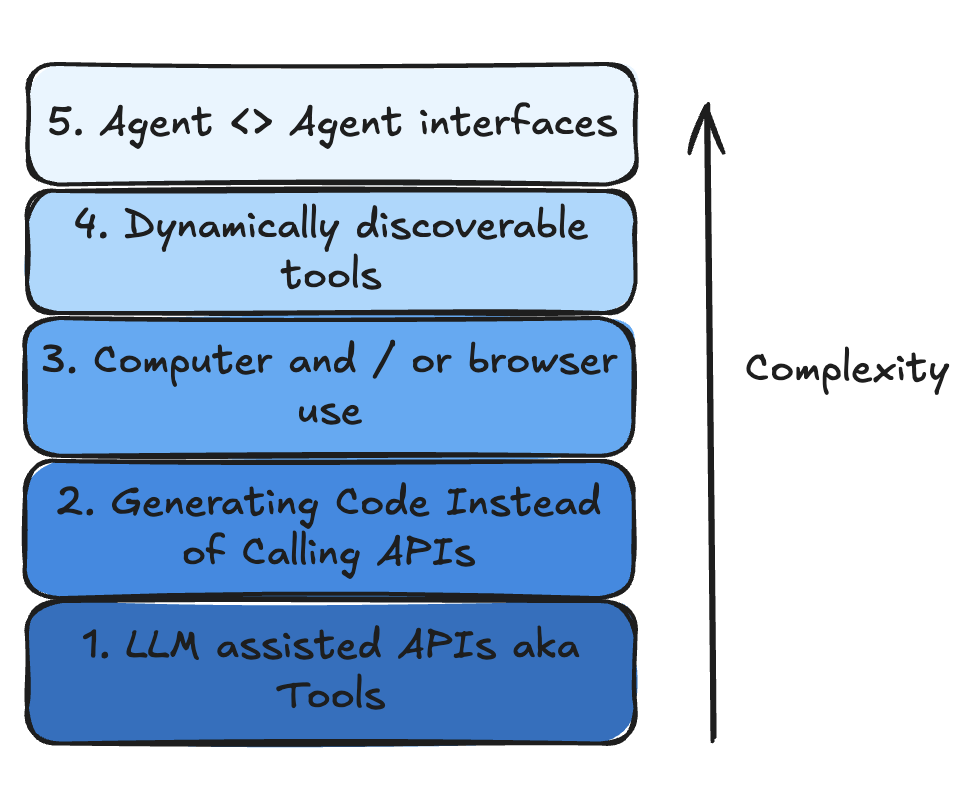
Understandably, some of the reaction to A2A has been that it isn’t clear whether it is needed (and if it is, whether it is needed yet) and how it fits together with MCP (↗). In particular, with tools and agents ultimately both being a way to get a task done and facing many of the same challenges (discovery, task definition, input / output definition, auth etc.), some people are questioning whether we need another protocol on top of MCP, or whether it is enough to just wrap agents in tools. We’ve been diving into A2A over the last couple of days and wanted to share our thoughts on these topics.
Agents vs Tools
Both agents and tools are mechanisms for achieving tasks, but they generally differ significantly in complexity, autonomy, and interaction patterns:
- Task Definition: Tools handle narrow, clearly defined tasks; agents handle broad, higher-level, open-ended goals.
- Autonomy: Tools just do what they’ve been programmed to do; agents act autonomously, breaking down goals and seeking additional info if needed.
- Input: Tools take structured input; agents understand natural language.
- Single Step vs Multi Step: Tools are generally single-shot, with a call either returning outputs or an error. Conversely, agents break a task down and work through it in multiple steps. This may involve the agent proactively reaching out to collect more information for the task, or even asking the user some clarifying questions.
- State: Tools are stateless; agents can build context over time.
- Length: Tools generally run quickly, with most APIs returning in less than a second; agents may work over minutes, hours, or days.
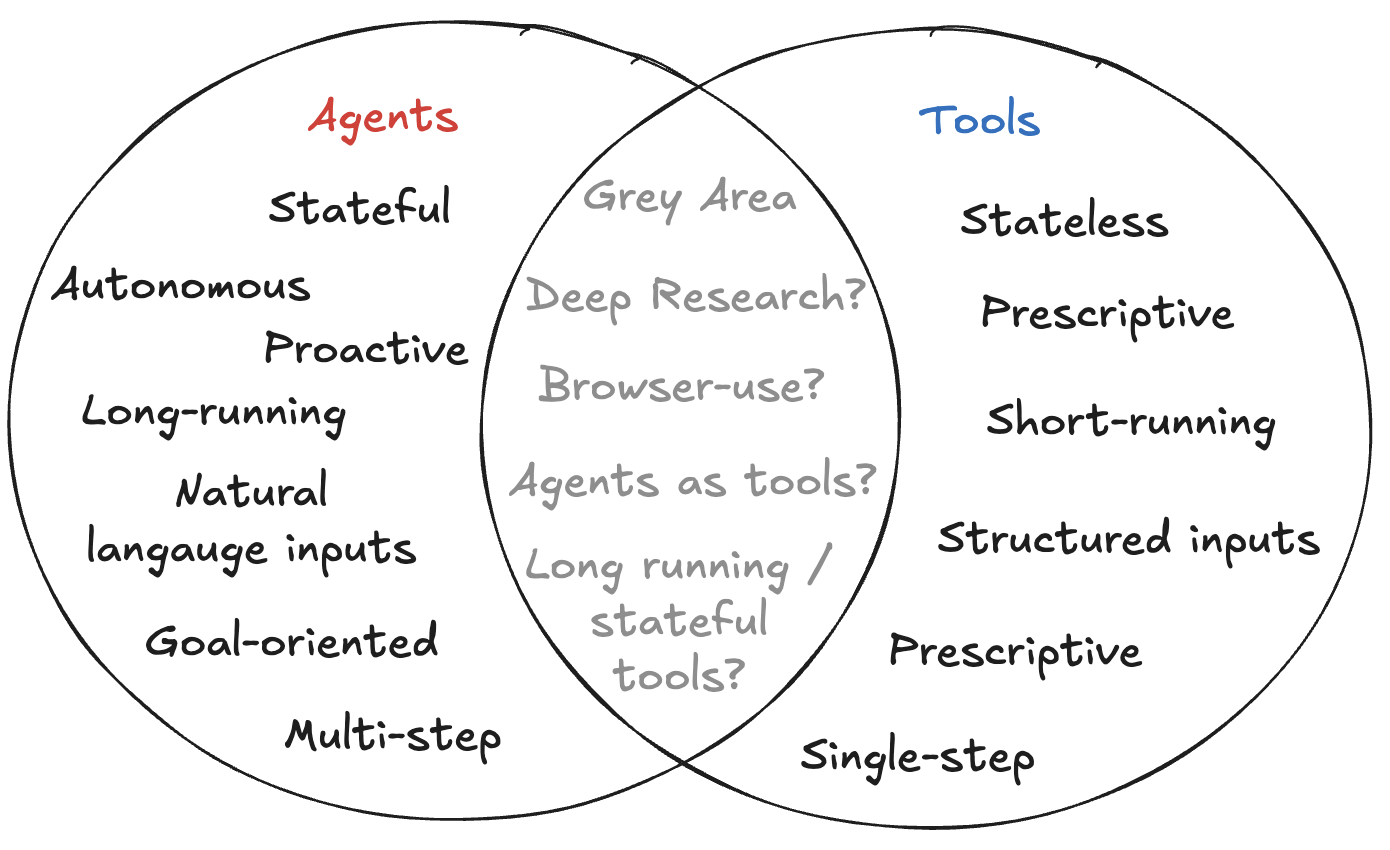
While this can seem a clear and natural divide, our work at Portia shows there's often a grey area between them:
- Browser Use (↗): Though agent-like in behavior (e.g., autonomous navigation via natural language), we’ve had success using browser tools in a single-turn, tool-like way to retrieve structured data.
- Deep Research: Some implementations behave like slower search tools, others like full agents asking clarifying questions. Sometimes the same implementation can display both, depending on the query.
- Agentic Tools: Tools can show agent-like traits: holding state (e.g., counters), running long processes (e.g., ML training), or even handling tasks that might require an agent in more complex scenarios (e.g. document retrieval).
- Single vs Multi step: Even the clearest distinction – single vs. multi-step interaction – isn’t absolute. Just as agents ask for additional information, tools throw errors detailing the info they need. Often the loop needed to handle both is the same.
With the distinction between the categories quite blurry, it certainly adds complexity to the ecosystem if you need different protocols for the different sides of the spectrum.
A2A & MCP
A2A (Agent-to-Agent) is a protocol designed for enabling autonomous agents to communicate, discover each other, and collaborate on tasks. Positioned at the agentic end of the spectrum, A2A focuses on agents that can take higher-level responsibility for executing tasks, compared to MCP which is more tool-oriented.
To demonstrate the difference, imagine booking a dinner using an agent. With MCP, a restaurant booking platform might expose tools such as ‘find_restaurants’ or ‘book_restaurant’. My agent must then use these tools to achieve the goal of organising dinner.
Conversely, with A2A, the restaurant booking platform provides an agent with a skill for finding and booking restaurants – a concept deliberately looser than a tool. The remote agent will then take control of the full task, including tracking its state and deciding when to communicate with my local agent and when to mark the task as complete.
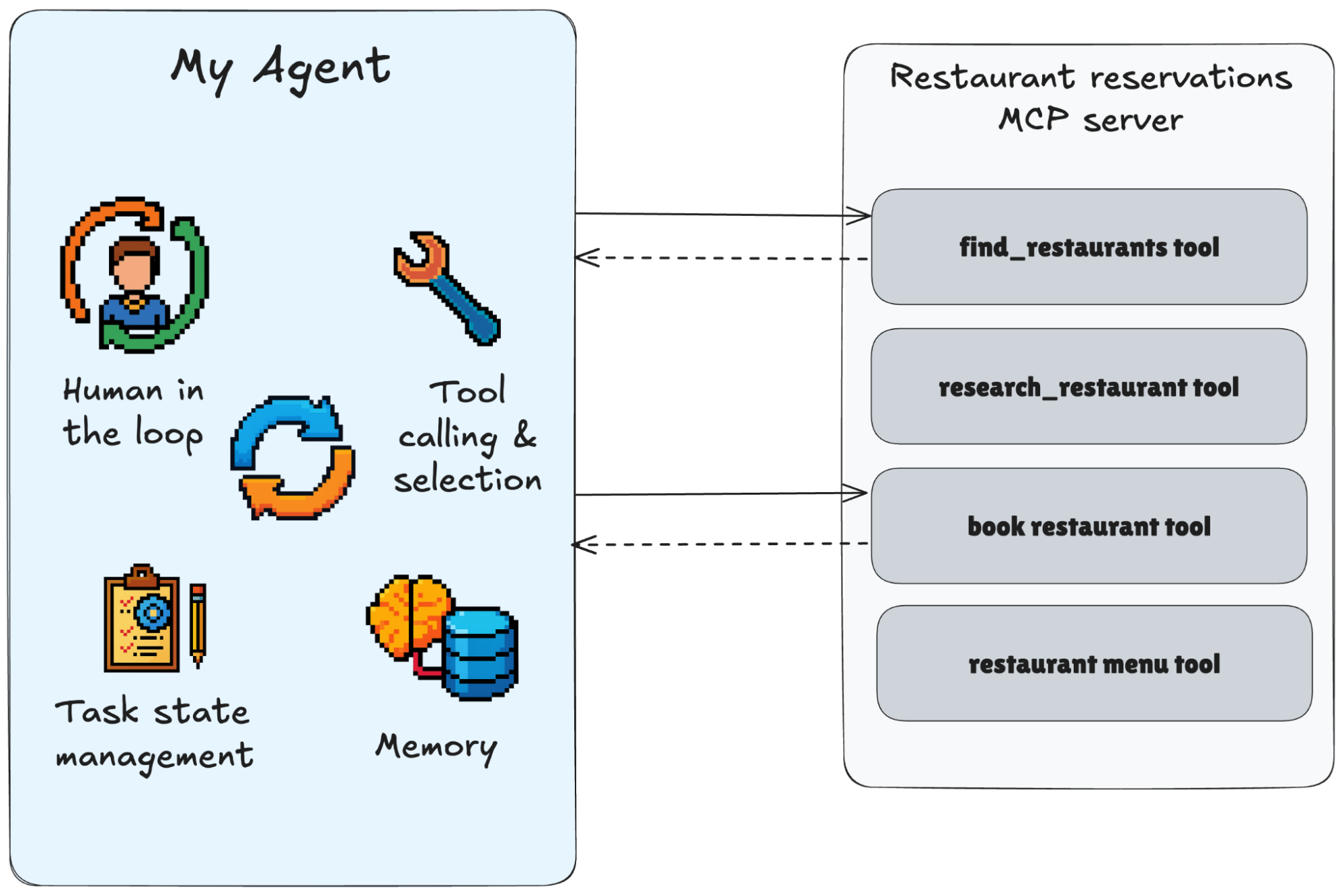
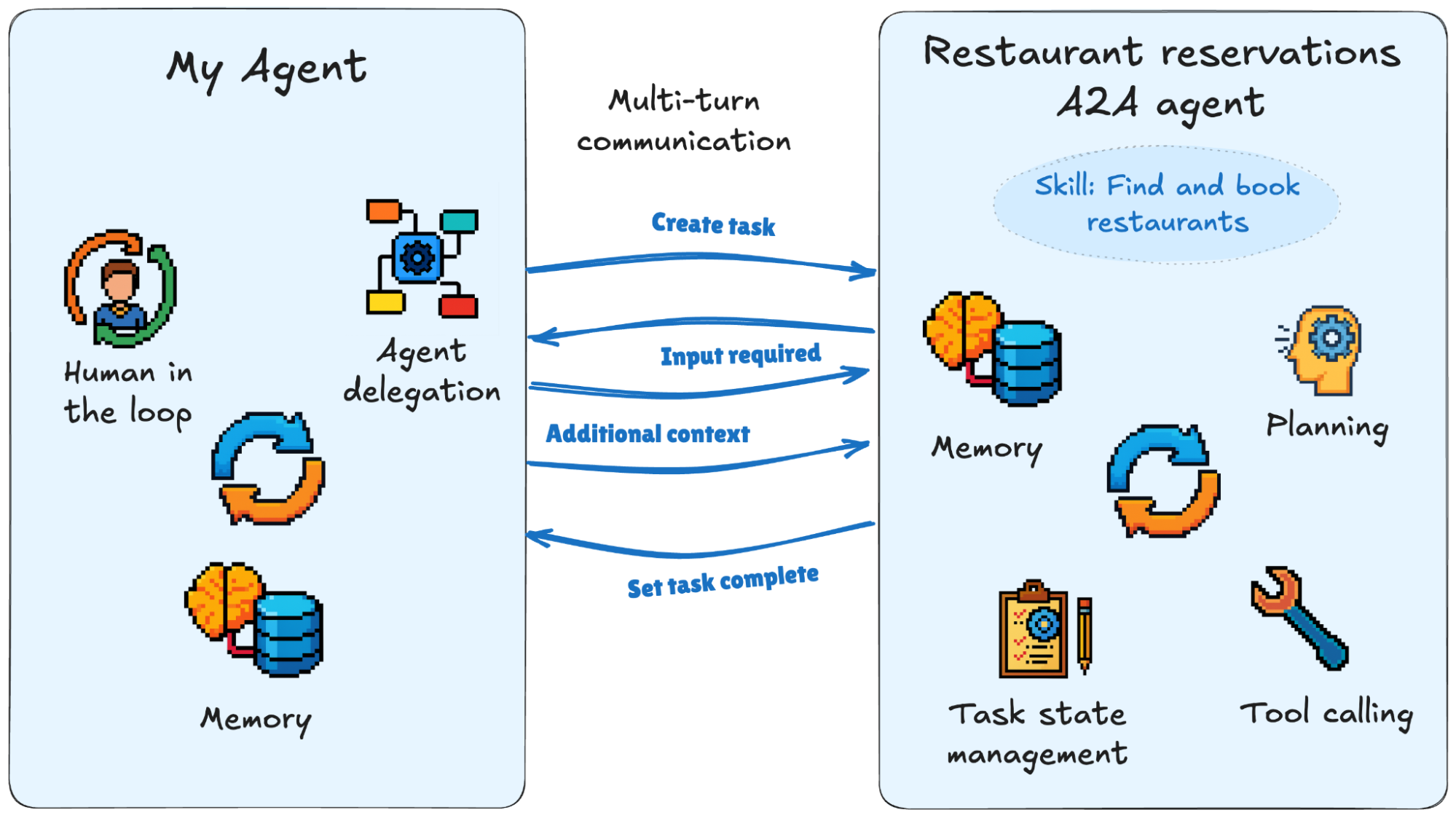
To dive a bit deeper, let’s take a look at the core components of A2A:
- Agent Description: A2A agents have JSON "agent cards" outlining skills, auth methods, and input/output formats. These are higher-level and less structured than MCP's task-focused tool descriptions.
- As the skills description within A2A is deliberately more vague, it will be interesting to see how people handle defining the boundary around what a particular agent can and can’t do.
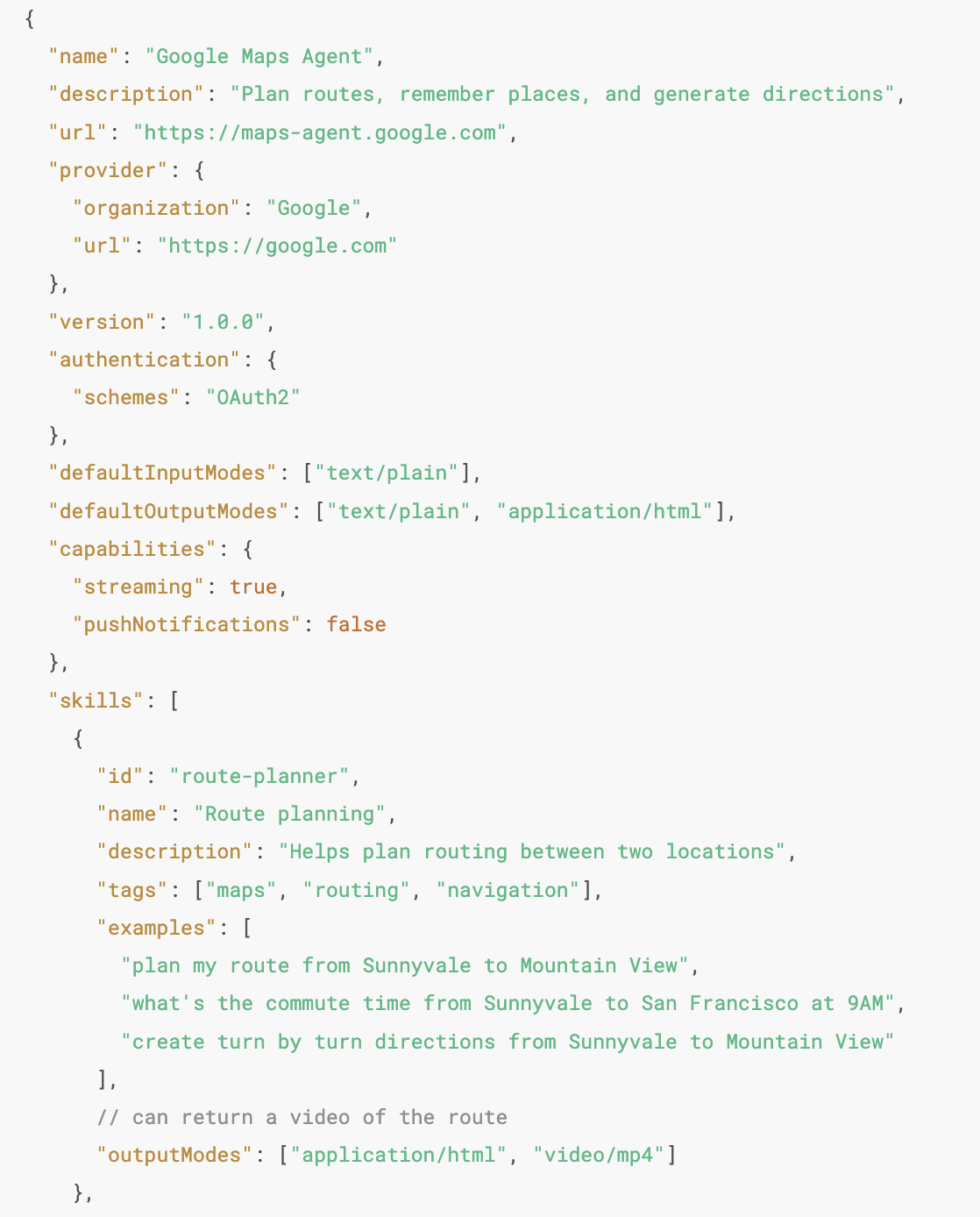
- Agent Discovery: Agents can be discovered via a well-known URL (
/.well-known/agent.json
). Registries are likely to be added, similar to MCP’s tool registries. - Multi-step Interactions: A2A supports long-running tasks through multi-message exchanges, allowing agents to schedule, negotiate, and send progress updates. MCP does not yet have support for this richness of multi-message exchanges.
- Offline Handling: As tasks are long-running and agents may not have a session open for the full duration, A2A has support for agents sending push notifications that are received later by the client (e.g. for task updates). This is not supported natively in MCP.
- Auth: A2A supports all OpenAPI auth schemes (e.g., API keys, OAuth2, JWTs), offering more flexibility than MCP’s OAuth2-only approach. However, this also means that my local agent needs to handle all of these auth schemes too.
- Outputs: Task results are called "artifacts". These are the equivalent to MCP’s tool outputs but with the key difference that artifacts are split into parts by default.
MCP vs A2A: Our Predictions
User pov | Agent::tools | Agent::MCP servers | Agent::Agent (A2A) |
---|---|---|---|
Capability discovery and selection | 🔴 Tools have to be manually added to agent. Selection is limited to LLM’s ability to cope with large tool sets. | 🟡 Tools are automatically discovered through MCP. Selection is still limited to LLM’s ability to cope with large tool sets. | 🟡Agent capabilities are advertised through their agent card. Once registries have been added to the protocol, agents will be discovered through a registry. |
Ease of interface to other systems | 🔴 Developer has to understand the other system’s API in order to manually write / select tools. | 🟡 Agent calls MCP tools with a single-step interaction. My agent needs to understand the external system to determine how to chain tool calls together to achieve a goal. | 🟢 My agent connects to a remote agent to access other systems’ capabilities. The remote agent determines how to use these capabilities to achieve my goal. |
Auth | 🔴 Developer has to implement their own auth on tools for the agent to use | 🟡 Auth (based on OAuth2) has recently been released, though is yet to be widely adopted | 🟡 Launches with all auth schemes supported by OpenAPI, though this means my agent will need to support whichever auth scheme is supported by the remote agent |
Task control & completion | 🟢 My agent has full control over how the task is performed, including deciding when it is complete. All output from the other system is accessible to my agent via tools, and my agent determines what information is retained during and after runtime | 🟢 As with simple tool usage, my agent has full control over the task, its completion, the output of tools and how information is retained. | 🟡 My agent relies on controlling the remote agent through negotiation and relies on the information sharing and retention decisions of the remote agent. It also relies on the remote agent to decide when a task is deemed complete or when further input is needed. |
A summary of the various communication schemes between agents and tools. Traffic light symbols give an assessment of how well each technology solves the user problem.
As discussed in our previous blog post (Beyond APIs (↗)), we believe agent-to-agent communication will eventually become widespread. This communication will be interactive, multi-turn and goal-oriented, rather than utilising single-shot, transactional, rigid APIs and tools that are common now. At Portia, we’ve built our clarifications architecture (↗) to handle this and it’s exciting to see the ecosystem progressing in this direction.
However, we do not foresee A2A getting the same rapid adoption of MCP. MCP addressed a clear, mainstream problem: enabling agents to interact with APIs. Agent builders wanted to move beyond simple chat or RAG systems without building custom tools for every API, while API providers wanted to support agents without adapting to every agent framework. MCP elegantly solved this MxN problem by allowing providers to repackage their existing APIs and documentation into an MCP server easily.
In contrast, agent-to-agent communication hasn’t yet become mainstream and is significantly more complex. Deploying an agent in front of an API introduces challenges like managing ambiguous multi-turn requests, maintaining state, handling offline clients, and gracefully resolving cascading errors. Additionally, with the distinction between tools and agents not clear cut, it adds complexity to the ecosystem to have different protocols for both.
Therefore, in the short-term we expect companies to continue to focus on MCP and we expect to see a growing usage of agents within tools. We then expect to see MCP evolve to handle these ‘agents in tools’ use-cases more natively and elegantly, leading to MCP covering all of the 'tool-agent’ spectrum.